Synthetic data, crafted by cutting-edge generative AI models, presents a fairer, safer, and faster alternative for data-driven enterprises. Despite its benefits, misconceptions persist, skewing public perception. This article aims to clarify these misunderstandings.
Myth 1: Synthetic Data Isn't Secure:
Contrary to common belief, synthetic data is distinct from anonymized data. It is created using sophisticated AI models that leverage deep learning techniques to analyze the statistical distributions of original datasets. These models, such as Generative Adversarial Networks (GANs) or Variational Autoencoders (VAEs) or Transformers generate new data instances that reflect the complex multivariate relationships found in the original data without retaining any direct ties to individual data points. As a result, synthetic data contains no personally identifiable information (PII), preserving the anonymity of the data source while maintaining its statistical validity.
In contrast, anonymized data often relies on methods like data masking or perturbation, which can still leave traces of PII that sophisticated adversaries could exploit. Synthetic data, however, by not mapping directly to real data points, remains robust against such privacy attacks. This is demonstrated by its resilience against various de-anonymization techniques such as linkage attacks, where anonymized data has historically been compromised (e.g., Netflix Prize, JP Morgan Chase datasets).
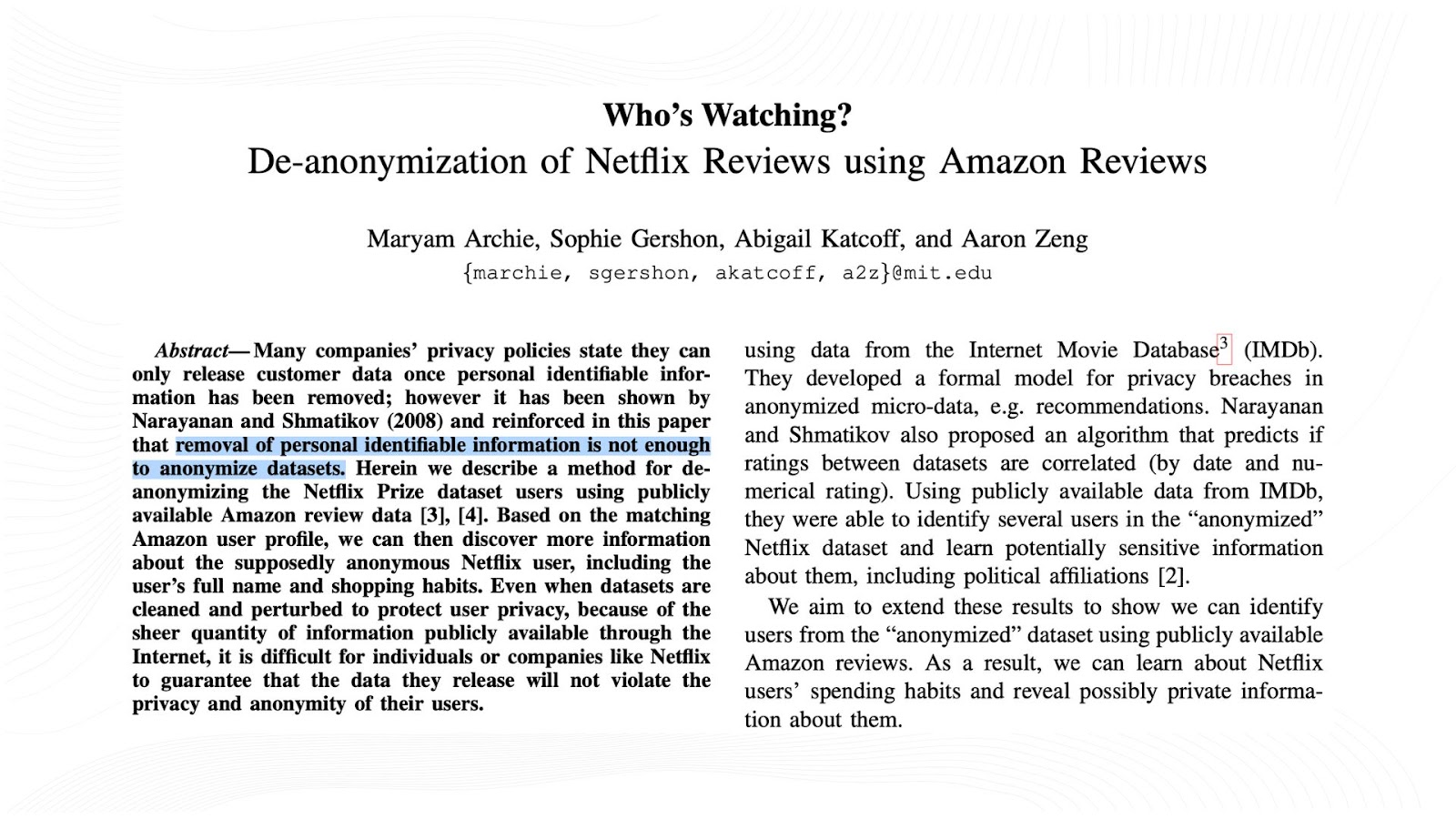

Furthermore, advanced synthetic data engines like Betterdata incorporate specific privacy metrics, including differential privacy guarantees, to quantitatively evaluate and enhance the data's resistance to potential privacy attacks. These metrics help organizations understand the effectiveness of synthetic data in protecting against privacy breaches, thus providing a more secure and reliable alternative for data utilization in sensitive domains.
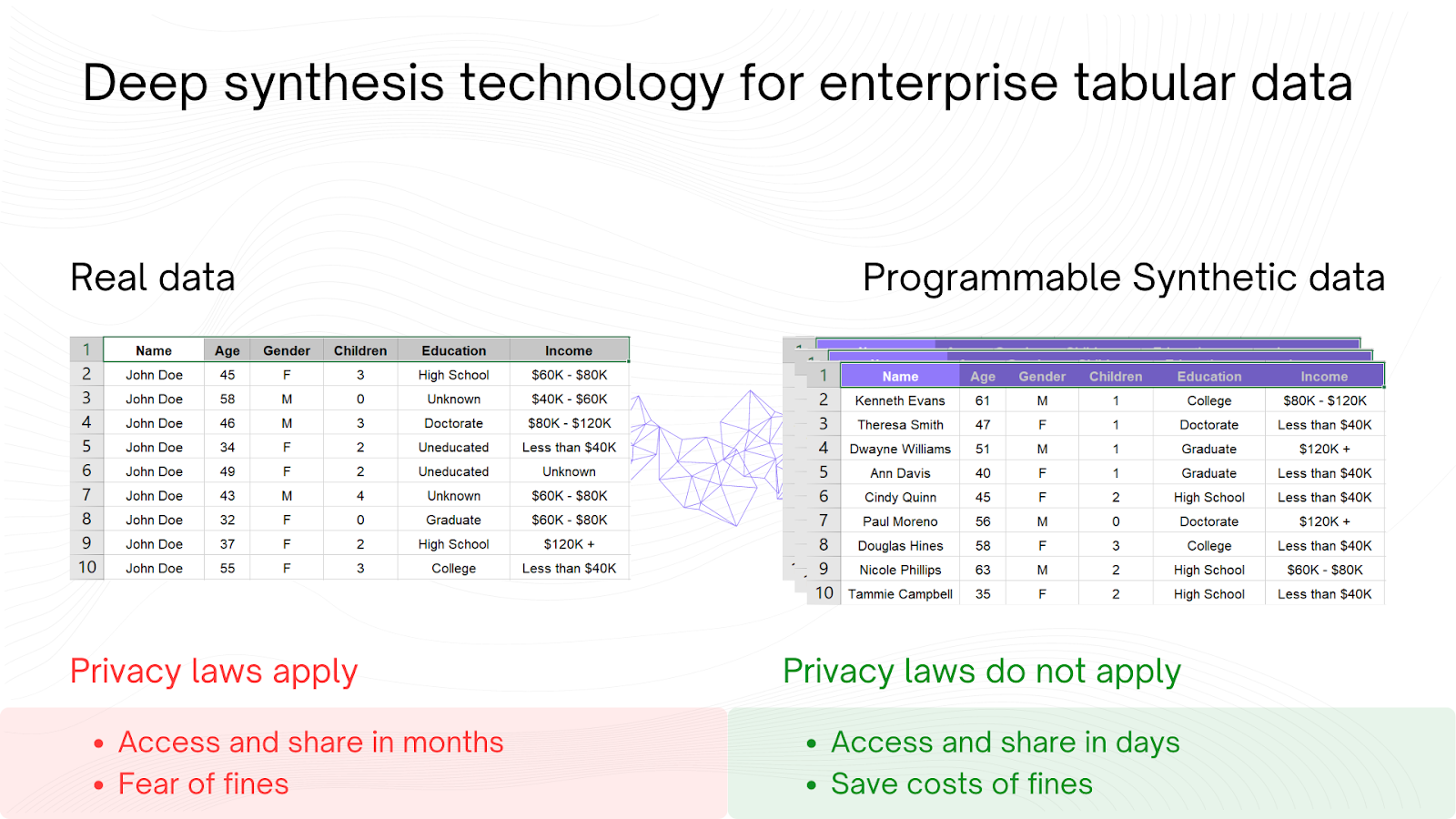
Myth 2: It’s Completely Fake and the Same as Anonymized Data:
Synthetic data is often misunderstood as merely an artificial or "fake" construct. However, this perspective overlooks its sophisticated generation process which involves state-of-the-art AI techniques like neural networks and machine learning algorithms that accurately model and reproduce the intricate statistical properties of original datasets. Unlike mere anonymization, which simply obscures or removes identifiers from data to prevent linkage to identities, synthetic data generation creates entirely new datasets that mimic the original's statistical depth without any actual data points from the original set. This ensures the utility of the data for analytical purposes without the risk of PII exposure, making it invaluable for training machine learning models where data privacy is crucial.
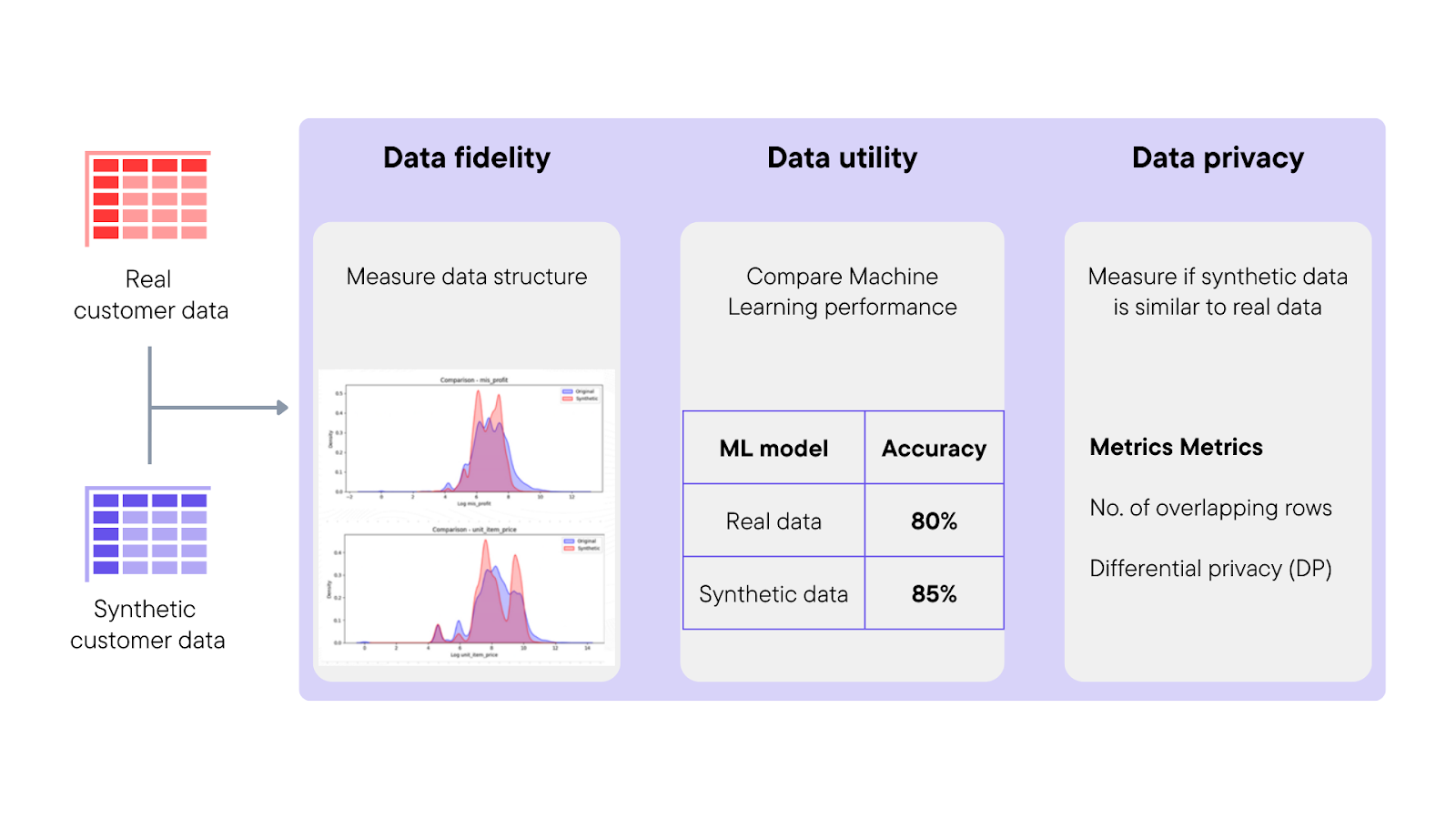
Myth 3: It’s Not Regulatory Compliant:
The generation of synthetic data is rigorously designed to align with current regulatory frameworks, particularly those focusing on data privacy such as GDPR, HIPAA, and others. By employing advanced algorithms, synthetic data is generated ensuring that no personally identifiable information is included. This adherence to privacy by design principles not only meets but often exceeds the requirements of privacy regulations, offering a safe harbor against potential data breaches and privacy violations. Moreover, as privacy regulations evolve and become more demanding, the flexibility and adaptability of synthetic data generation processes allow continuous compliance without the need for frequent process overhauls. This reduces the operational burden associated with maintaining data privacy standards, helping organizations navigate the complex landscape of data privacy with greater ease and less risk.
Synthetic data is not ‘real’ data created naturally through real-world events, rather it is ‘artificial’ data, generated using algorithms. The benefit of using synthetic data is that it simulates real data without identifying specific individuals, therefore as long as no real individuals can be identified from the synthetic data, data protection obligations, such as GDPR, do not apply." - FCA
Myth 4: Synthetic Data Generation is Expensive:
The notion that synthetic data generation is prohibitively expensive stems from a misunderstanding of its long-term financial benefits. Initially, the investment in advanced generative technologies and the necessary computational resources might seem substantial compared to traditional data anonymization methods. However, synthetic data platforms leverage sophisticated algorithms such as GANs (Generative Adversarial Networks) or Transformers to create data that is free from privacy concerns and tailored to specific analytic needs. This precision allows organizations to conduct research, develop products, and train AI models with datasets that are both diverse and reflective of real-world complexities without risking PII exposure.
Furthermore, traditional data anonymization often needs to improve in protecting against modern de-anonymization techniques, potentially leading to breaches that incur hefty fines and reputational damage. In contrast, synthetic data eliminates these risks by design, ensuring compliance with stringent data protection regulations like GDPR. This proactive approach to data privacy safeguards against financial penalties and reduces the need for costly data security measures typically associated with PII management.
Companies like Betterdata harness these advanced capabilities to provide cost-effective solutions outperform traditional methods. By integrating comprehensive compliance, privacy protection, and high-fidelity data utility, synthetic data generation proves to be an economically viable option, especially when considering the indirect costs of data breaches and regulatory non-compliance. This makes synthetic data a strategic investment that, while appearing expensive upfront, offers substantial cost savings and competitive advantages over time.
If you found this helpful, also read: Synthetic Data vs Data Anonymization. Which is Better?
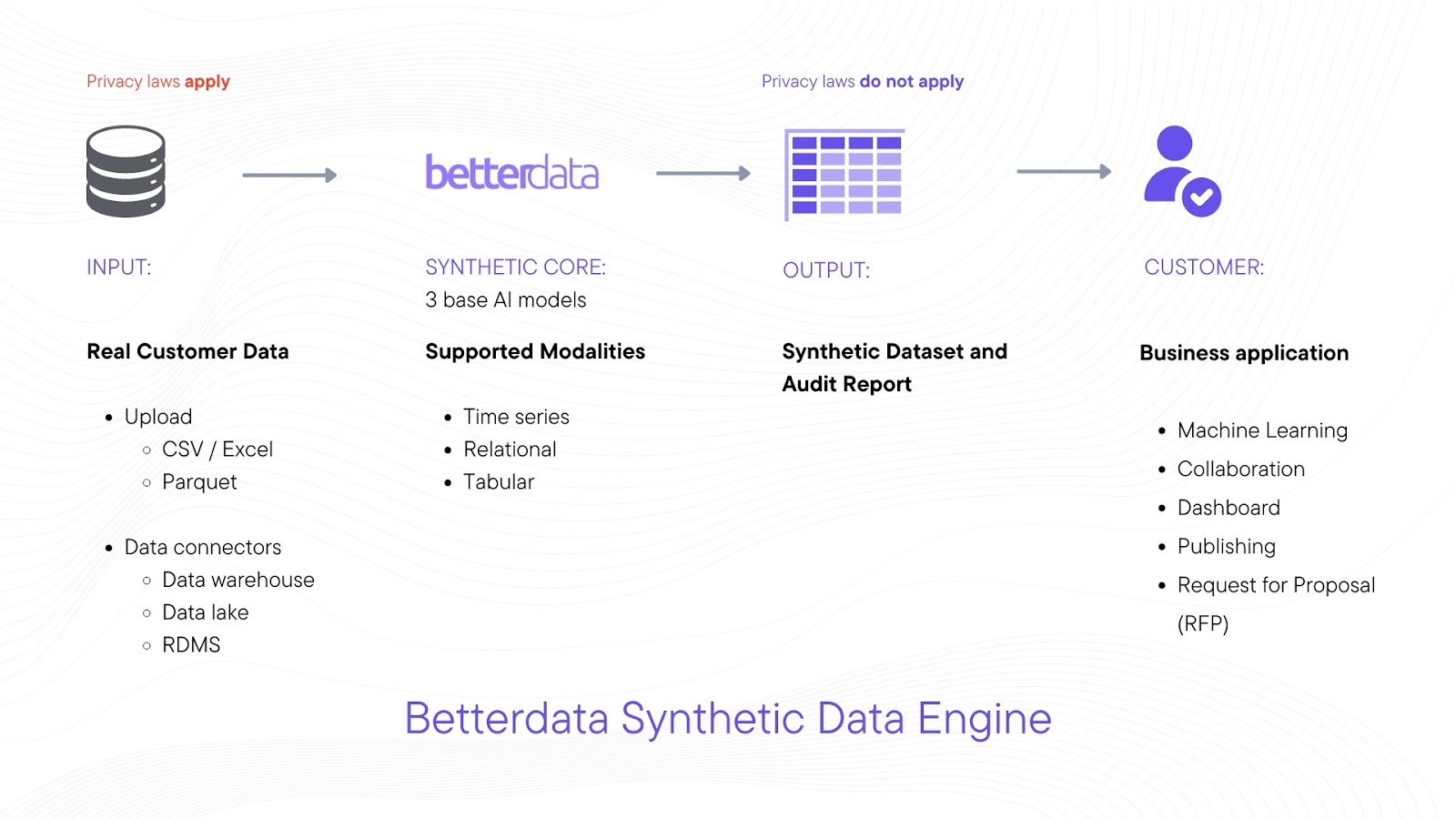
Conclusion:
In conclusion, synthetic data, generated by advanced generative AI models, offers a robust and secure alternative to traditional anonymized data. It effectively addresses common misconceptions by providing data free from personally identifiable information (PII) while retaining the statistical integrity of the original datasets. Unlike anonymized data, which is susceptible to de-anonymization attacks, synthetic data is constructed from learned statistical patterns, ensuring safety from such vulnerabilities. Furthermore, synthetic data is fully compliant with regulatory standards, safeguarding digital privacy without the risk of PII leakage. Its generation, although perceived as expensive, proves cost-effective by reducing operational difficulties and the risk of regulatory penalties. Overall, synthetic data stands out as a fairer, safer, and more efficient option for data-driven companies looking to leverage the potential of AI without compromising security and compliance.