‘Data Driven’ is probably a word you have heard a thousand times within the last business quarter and rightly so. The world is all about data now. With advancements in technology, intense product personalization, and extreme market competitiveness, every company is now placing their bets on data to guide them on what, when, and where to make the next big business move—making data a tradeable commodity on its own. The data monetization market in 2024 is valued at 4.17 billion $ and is predicted to grow up to 10.35 billion $ by 2030.

This presents a unique opportunity for organizations with excess data to create alternate revenue streams through data monetization that is if they can bypass stringent data privacy and protection laws, and maintain data utility, value, and security by applying extensive data anonymization techniques which in recent time have not been proven to be as effective as they once were. But we are here to discuss solutions, not problems. And the solution is the much-talked-about synthetic data.
1. What is Synthetic Data:
Synthetic data is created through advanced AI models that use deep learning methods to mirror the statistical characteristics of real-world data, all while avoiding any replication of personal information. Techniques like Generative Adversarial Networks (GANs), Variational Autoencoders (VAEs), and Transformers are used to analyze the statistical patterns of original datasets that recreate the complex multivariate relationships present in the original data, ensuring no direct connection to individual data points protecting the anonymity of the data sources while preserving its statistical integrity which is why synthetic data can be used effectively for data monetization.
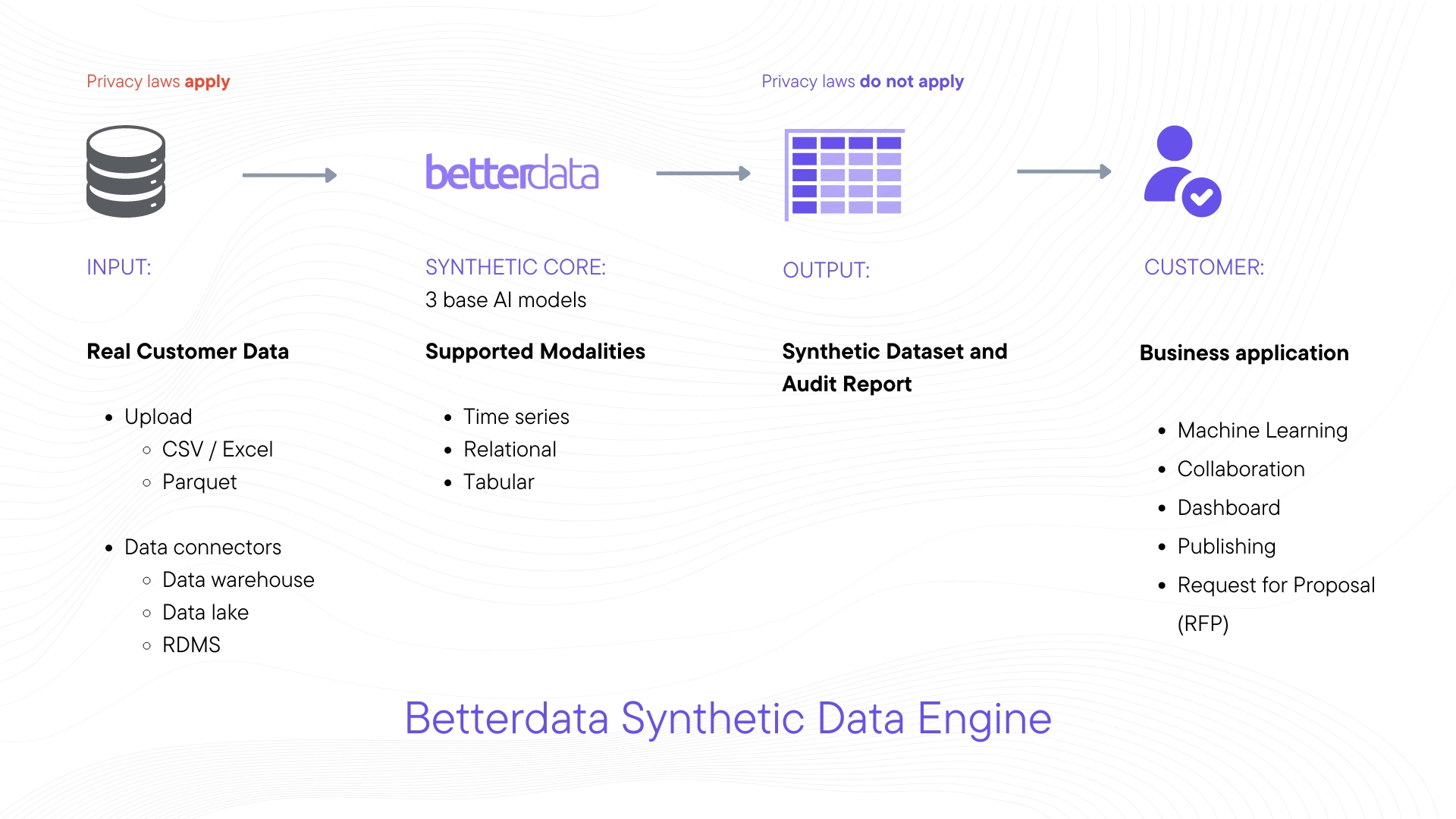
2. Data Monetization Opportunities with Synthetic Data:
a. Data as a Service (DaaS):
Offering synthetic data as a service allows businesses to provide clients with customized datasets for various purposes such as testing, machine learning model training, or research.
- Customization: Businesses can tailor synthetic data to meet specific industry needs. For example, healthcare companies may require data that mimics patient records without violating HIPAA regulations.
- Scalability: Using cloud-based platforms, businesses can generate and distribute synthetic datasets at scale, ensuring that clients receive the data quickly and efficiently.
- Data Generation Techniques: Techniques like GANs and VAEs can generate highly realistic data that preserves the statistical properties of the original datasets, ensuring utility for clients while maintaining privacy.
Use Case: Financial Institutions can use synthetic data to test a new fraud detection algorithm, ensuring it performs well under various scenarios without risking exposure to real client data.
b. Enhanced Product Development:
Companies can utilize synthetic data to enhance their products and services. In the software industry, synthetic data is invaluable for testing applications under diverse conditions. They can acquire this data through data marketplaces which allows other organizations to monetize their data.
- Simulation and Testing: Synthetic data can be used to simulate rare but critical scenarios that may not be present in real-world datasets. This helps in identifying potential issues and improving product robustness.
- Iterative Development: By generating synthetic data on demand, companies can continuously test and refine their products throughout the development cycle.
- Cross-Industry Applications: Beyond software, industries like automotive (for autonomous vehicle testing) and manufacturing (for predictive maintenance) can benefit from synthetic data.
Use Case: A software company uses synthetic user interaction data to test the performance of a new feature across various devices and operating systems, leading to a more reliable and user-friendly application.
c. Collaborative Partnerships:
Synthetic data facilitates safe data sharing between organizations, enabling collaborative research and development without compromising sensitive information.
- Secure Data Exchange: Using synthetic data ensures that no PII is shared, reducing the risk of data breaches and ensuring compliance with data protection regulations.
- Data Integration: Synthetic data can be integrated with other datasets to provide comprehensive insights while maintaining privacy.
- API Access: Providing API access to synthetic data can streamline collaboration, allowing partners to access and utilize the data seamlessly.
Use Case: Two pharmaceutical companies collaborate on drug development by sharing synthetic patient data, accelerating research without violating patient confidentiality.
d. Market Insights and Analytics:
Organizations can monetize synthetic datasets by selling them for market insights and analytics. These datasets can help businesses identify trends, preferences, and patterns.
- Big Data Analytics: Synthetic data can be generated in large volumes, enabling comprehensive market analysis.
- Trend Analysis: Advanced analytics tools can process synthetic data to uncover emerging trends and consumer behaviors.
- Anonymity and Validity: Synthetic data ensures that market insights are derived without compromising the anonymity of the original data sources.
Use Case: A marketing firm sells synthetic consumer data to retail companies, helping them to tailor their marketing strategies and product offerings based on identified trends and preferences.
e. Training AI and ML Models:
The demand for high-quality data to train AI and machine learning models is ever-growing. Synthetic data can meet this need, offering diverse and extensive datasets that enhance model accuracy and performance.
- Data Diversity: Synthetic data can be generated to cover a wide range of scenarios, improving the robustness of AI models.
- Bias Mitigation: By carefully generating synthetic data, biases present in real-world data can be reduced or eliminated, leading to fairer AI models.
- Continuous Improvement: Synthetic data allows for ongoing training and improvement of AI models, ensuring they remain accurate and effective.
Use Case: An AI startup uses synthetic data to train a machine learning model for image recognition, achieving high accuracy without the need for large volumes of labelled real-world data.
Read Also: Use Cases for Synthetic Data Across Industries

3. Use Cases of Data Monetization through Synthetic Data:
a. Autonomous Vehicle Development:
Scenario:
An autonomous vehicle (AV) company is developing new software to improve the safety and efficiency of its self-driving cars. Real-world data collection from sensors and cameras is time-consuming, expensive, and can involve privacy concerns.
Problem:
The AV company needs large volumes of diverse and high-quality data to train and validate its machine learning models, but using real-world data presents challenges related to data privacy, collection costs, and coverage of all possible driving scenarios.
Solution: Synthetic Data as a Service:
- Customization: The synthetic data provider customizes datasets to include various driving scenarios such as urban streets, highways, different weather conditions, and pedestrian behaviors. This ensures the training data covers a wide range of situations that the AV might encounter
- Scalability: Using cloud-based platforms, the provider generates and distributes these synthetic datasets at scale, allowing the AV company to receive large volumes of data quickly and efficiently.
- Data Generation Techniques: Advanced techniques like GANs and VAEs are employed to create realistic sensor and camera data that mimic real-world driving environments. The synthetic data maintains the statistical properties and variability needed for effective model training while ensuring no real-world data privacy issues.
Benefits:
- Cost-Effective: Reduces the cost of data collection since generating synthetic data is cheaper than gathering real-world data.
- Comprehensive Coverage: Ensures the AV's machine learning models are trained on a wide range of scenarios, improving their robustness and safety.
- Privacy Compliance: Eliminates concerns about capturing and using real people's data, ensuring privacy and compliance with regulations.
Implementation Example:
The AV company subscribes to a synthetic data service, specifying the types of scenarios and conditions needed. The service generates and delivers the synthetic datasets, which the company uses to train and validate its machine learning models. Over time, as the AV software improves, the company can request new datasets to test and refine its algorithms further, ensuring continuous improvement and safety of its autonomous vehicles.
b. Retail Fashion Brand:
Scenario:
A retail fashion brand wants to understand the latest trends in consumer preferences to adjust its product lines and marketing campaigns accordingly.
Problem:
The brand struggles with acquiring detailed consumer data due to privacy laws and the high cost of real data collection.
Solution: Synthetic Consumer Data:
- Data Acquisition: The marketing firm generates synthetic consumer data based on historical data patterns and emerging trends. This data includes simulated consumer profiles, purchasing habits, and interaction histories.
- Advanced Analytics: Using advanced analytics tools, the firm processes the synthetic data to uncover emerging fashion trends, such as the increasing popularity of sustainable materials or specific color preferences.
- Insights Delivery: The firm provides the retail fashion brand with detailed reports and dashboards showcasing trends and consumer behavior insights. The synthetic data highlights patterns like peak shopping times, preferred product categories, and demographic segments with the highest engagement.
Benefits:
- Informed Decision-Making: The fashion brand can tailor its product lines to align with current trends, ensuring better market fit and customer satisfaction.
- Targeted Marketing: Insights from the synthetic data allow the brand to design more effective marketing campaigns, focusing on consumer segments most likely to engage with their products.
- Privacy Compliance: By using synthetic data, the firm ensures that all insights are derived without exposing any real consumer information, maintaining strict compliance with privacy regulations.
Implementation Example:
The retail fashion brand subscribes to the marketing firm's synthetic data service. The firm generates and delivers synthetic consumer datasets, which the brand analyzes to identify key trends and preferences. Based on these insights, the brand adjusts its product offerings and marketing strategies, increasing sales and customer engagement. Over time, the brand continues to use synthetic data to stay ahead of market trends and maintain a competitive edge.
4. Conclusion:
And there you have it—your ticket to creating new revenue streams for your business through data monetization with data synthetic data. Synthetic data’s versatility combined with its privacy-protecting quality makes it a front contender for any company looking to add value to its business.