Less is NOT more. This is the reality of today’s business world when it comes to customer engagement and satisfaction. AI while still being adopted by the world has shown immense potential to transform customer-facing operations for better customer experience with one ex exception. The data required to train AI/ML algorithms is massive and leaves private and sensitive customer data at risk of exposure.
In this blog, we will talk about one very simple yet highly risky step to train AI/ML models, which is migrating sensitive customer data to the cloud and how synthetic data makes it safer, faster, and easier to do so.
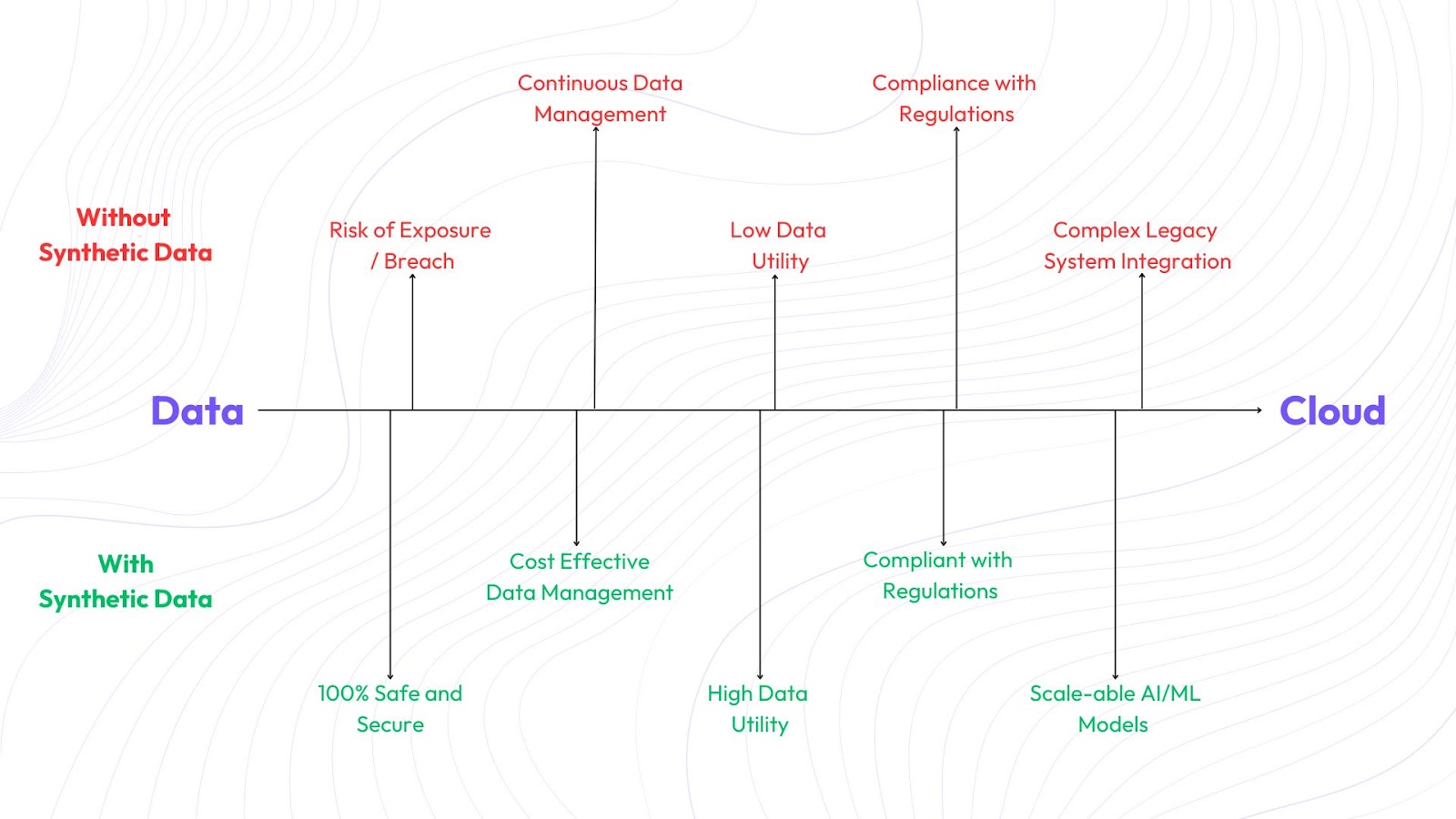
1. Challenges in Cloud Migration for Banks and Financial Institutions:
a. Data Security and Privacy:
Protecting sensitive customer data during and after cloud migration is one of the foremost concerns for financial institutions and banks, that deal with vast amounts of confidential information. During migration, data can be vulnerable to cyberattacks, unauthorized access, and breaches. Therefore, ensuring that customer data is encrypted both in transit and at rest is essential. Furthermore, the organizations must comply with stringent local and international privacy regulations such as PDPC, GDPR, CCPA, and other region-specific laws. Failure to comply can result in hefty fines and reputational damage.
b. Data Retention:
Data retention policies require careful management to ensure that real customer data is only kept for as long as necessary. After cloud migration, real data must be deleted by legal retention periods, which vary by jurisdiction. This process requires precise tracking of data usage, retention timelines, and automated deletion processes to avoid unintentional data hoarding. Failure to delete data within the required timeframe can lead to regulatory violations and potential security vulnerabilities.
c. Data Utility:
For AI/ML models to function effectively, they need high-quality data that reflects real-world scenarios. However, when banks are unable to use real customer data due to privacy regulations, maintaining the utility of data becomes a challenge.
d. Regulatory Compliance Across Multiple Jurisdictions:
Banks often operate across different regions, each with its regulatory framework. Ensuring compliance with varying data protection laws across jurisdictions adds complexity to cloud migration, as the bank must navigate diverse data handling, transfer, and retention regulations.
e. Integration with Legacy Systems:
Many tier 1 banks still rely on legacy systems that are deeply ingrained in their operations. Migrating to the cloud requires seamless integration with these legacy systems, which can be difficult due to outdated technology and data formats. Ensuring smooth interoperability between cloud-based AI/ML systems and existing infrastructure is a significant challenge.
2. Synthetic Data Makes Cloud Migration Simpler and Safer:
a. Cloud Migration with Synthetic Data
Before migrating to the cloud, synthetic datasets are created on-premises. These synthetic datasets mirror real customer data, allowing the bank to move to the cloud without transferring sensitive information. This approach ensures compliance with stringent security and privacy laws while maintaining data utility for AI/ML model training.
b. Scalable AI/ML Training in the Cloud
Once the synthetic data is in the cloud, the bank can scale its AI/ML training efforts. The synthetic data allows AI/ML models to be trained on large datasets, resulting in more accurate and personalized banking services. Continuous monitoring and validation ensure that model performance remains accurate and reliable.
c. Automated Data Deletion
One of the critical advantages of synthetic data is the ease of data deletion. After the synthetic data is generated, the real customer data can be securely deleted from both on-premises and cloud storage. Automated processes can ensure ongoing compliance with data deletion policies, reducing the risk of data breaches and regulatory penalties.
d. Enhanced Data Privacy and Anonymity
Synthetic data offers a significant advantage in protecting customer privacy. Unlike traditional anonymization techniques, which can still expose data to re-identification risks, synthetic data eliminates these concerns by not containing any actual customer information. By generating synthetic datasets that maintain the statistical properties of real data, the bank can utilize this data for AI/ML model training without any risk of re-identification, ensuring full compliance with data privacy regulations. This enhanced privacy protection builds trust with customers and regulators alike, demonstrating the bank's commitment to safeguarding sensitive information.
e. Cost-Effective Data Management
Synthetic data significantly reduces the cost burden associated with data storage and management. By generating synthetic datasets, the bank can minimize the amount of real data that needs to be stored, encrypted, and protected. This leads to lower storage costs, reduced data transfer expenses, and simplified data governance procedures. Additionally, synthetic data reduces the need for costly anonymization and encryption processes, which are required when handling real data. Overall, the use of synthetic data creates a more cost-effective approach to managing data while maintaining high standards of privacy and security.
Conclusion
As banks navigate the complexities of cloud migration and AI/ML training, synthetic data offers a powerful solution. It not only safeguards sensitive customer information but also enhances AI/ML capabilities, reduces costs, and ensures compliance with privacy regulations. For financial institutions looking to stay ahead in a data-driven world, synthetic data is the key to unlocking the full potential of AI/ML.