Machine Learning needs data. A lot of it. A general rule of thumb in machine learning is that for a dataset with 10 features (columns), you typically need at least 100 samples (rows) to ensure the algorithm can effectively learn the relationships and patterns within the data.
Large-scale machine learning models, such as Large Language Models (LLMs), Deep Generative Models (DGMs), Generative Adversarial Networks (GANs), and others, often require vast amounts of data for training, ranging from thousands to millions of data points.
This is necessary to capture complex patterns, ensure robust generalization, and achieve high performance across diverse tasks and applications. However, data is not so easily acquired or used,
❌ Real data often suffers from scarcity, making it difficult for robust model training.
❌ Low data quality such as noise, inconsistencies, and errors degrades model performance.
❌ Biased or imbalanced datasets lead to unfair or skewed outcomes, compromising fairness.
❌ Handling sensitive or personal data requires strict compliance with regulations like GDPR, PDPC, etc.
❌ Data collection and annotation are costly and time-intensive.
❌ Ensuring data remains relevant and up-to-date for the problem domain is critical.
❌ Security risks, such as breaches or unauthorized access, threaten data integrity during storage and processing.
Impact on Model Performance:
The quality of a machine learning model depends on the data it is trained on. As pointed out above data is not a readily available resource that enterprises can fit into model training at any given time of the day. This limitation degrades the model performance where it is not able to learn patterns and insights generating sub-optimal results depicted in the Illustration below.
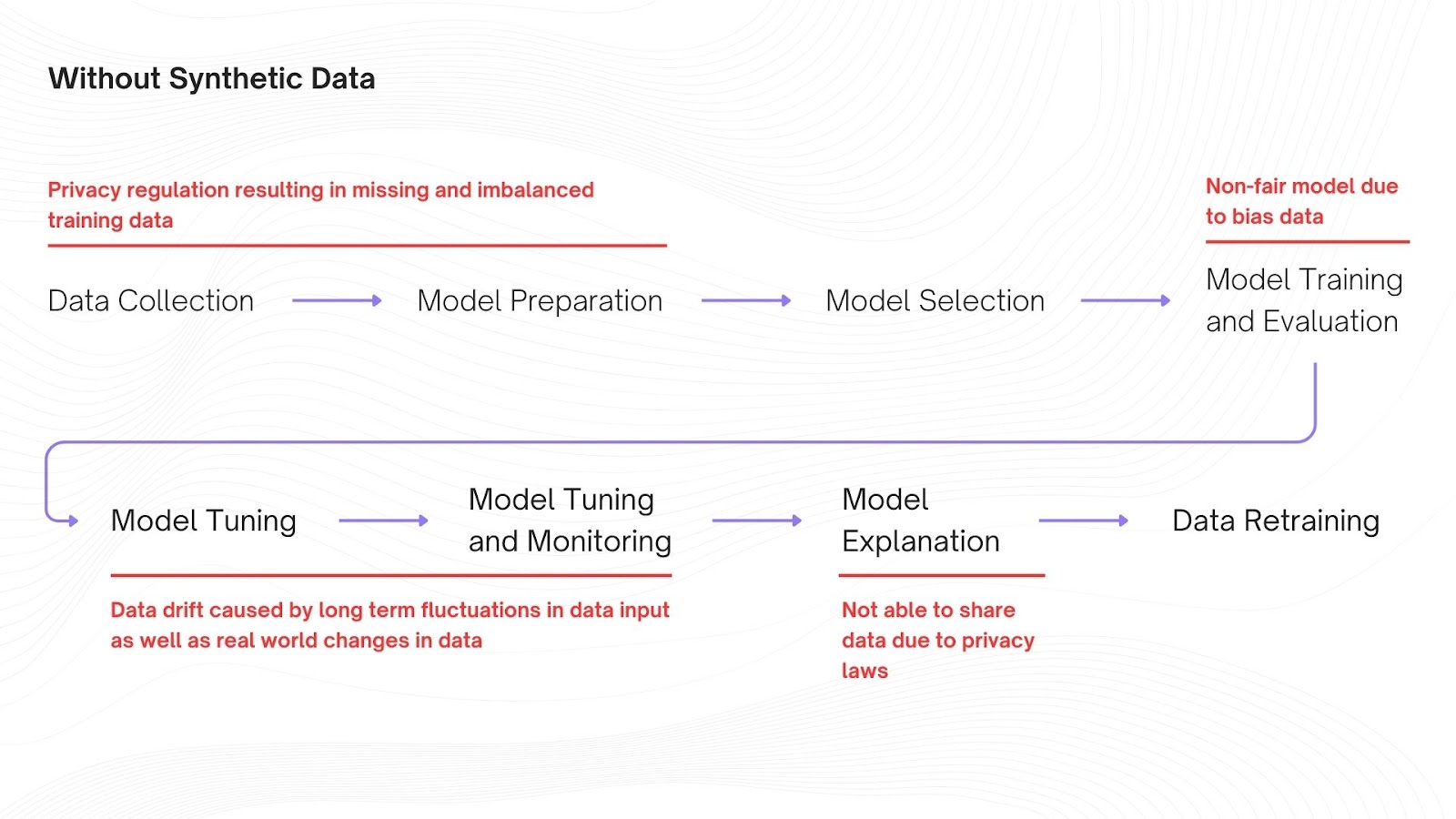
Improving ML Model Performance with Synthetic Data:
✔ With synthetic data augmentation you can expand the size, diversity and variability of an existing datasets by generating additional synthetic data allowing ML models to generalize better over a large range of scenarios.
✔ Synthetic data enhancement enables you to improve the data quality and utility by adding new features, filling in missing values, reducing gaps, correcting imbalances, etc. improving domain coverage.
✔ Synthetic data removes bias in training data allowing ML models to train on a fair, balanced, unbiased and comprehensive dataset.
✔ Synthetic data is artificially generated therefore can be scaled easily and quickly to meet the data needs of large scale ML models.
✔ You can customize synthetic datasets to meet specific requirements or scenarios to train a ML model for a specific task.
✔ Since synthetic data is not anonymized it does preserve data utility and accurately represents real data statistics and patterns.
✔ Synthetic data does not contain PIIs making it compliant with data privacy laws.
✔ Synthetic data can be shared quickly and safely internally and externally without compromising data privacy enabling quick feedback, model validation and data analysis.

While using real data is everyone’s dream. It is in fact only a dream. The reality is that you can’t use real data at least without anonymizing it. But that has its problems. Anonymized data has been proven to be ineffective in training large ML models because of the amount of PII that it masks, encrypts, or destroys degrading data utility. Synthetic data is a more viable solution for scaling ML models with high quality, representative and secure training data which can be generated and augmented on demand quickly saving both time and money.