In today’s rapidly evolving digital landscape, privacy has become a critical concern for organizations globally. As data scientists, privacy consultants, and top management, understanding the nuances of privacy technologies is essential for ensuring data security and compliance while leveraging the power of AI and machine learning.
In a recent panel discussion on the impact of AI on data privacy at the PDPA International Conference 2024, Co-Founder and CEO of Betterdata Dr. Uzair Javaid, a leading expert in synthetic data and privacy engineering, provided an in-depth analysis of various privacy technologies and their applications. His insights shed light on how organizations can navigate the complex world of data privacy while maximizing the utility of their data.
1. Two Words to Summarize Privacy Technologies: Adding Noise
At the core of privacy technologies lies a simple yet powerful concept: adding noise. While the methods of adding noise may differ, the fundamental goal remains the same—to protect sensitive information without compromising the utility of the data.
Over the past 20 years, data anonymization has been a widely adopted practice. This technique involves destroying or masking specific pieces of information within a dataset to protect privacy while retaining some level of utility. A simple way to understand anonymization is to cover half of your face with your hand. While a new observer might not recognize you, they can still discern that there is a face behind the hand. Similarly, anonymization obscures certain data points to protect individual identities.
Despite its widespread use, anonymization has its limitations, especially in the era of advanced AI and machine learning. As privacy concerns have grown, so too has the need for more robust and innovative solutions.
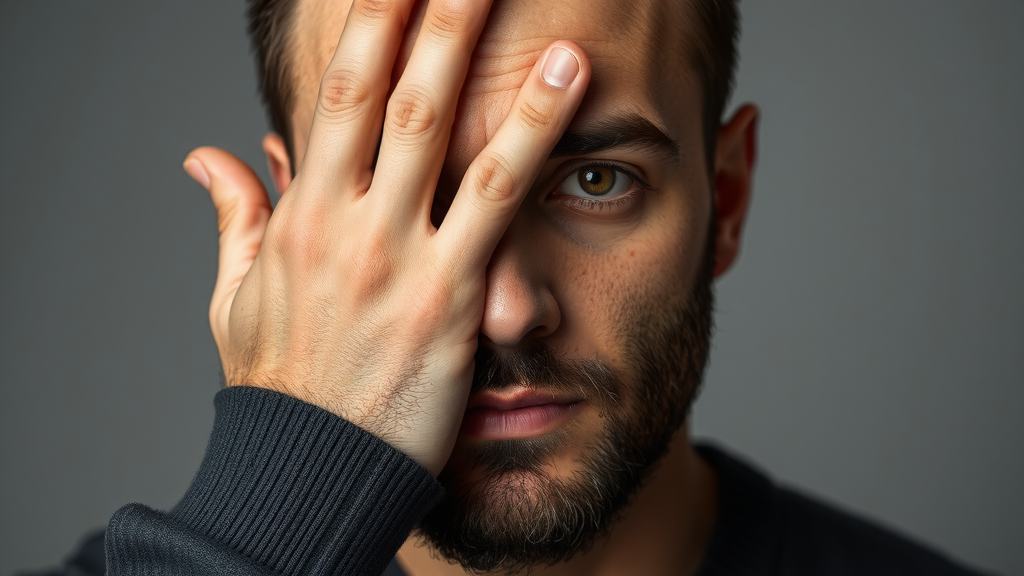
2. The Rise of Encryption-Based Technologies:
In the last decade, encryption-based technologies have gained traction, offering new ways to process and analyze data securely. Techniques such as homomorphic encryption, private set intersection, secure multiparty computation, and zero-knowledge proofs have emerged as key players in this space. These technologies enable data to be encrypted while still allowing for certain types of analysis, ensuring that sensitive information remains protected.
However, encryption is not without its challenges. While it provides robust privacy protection, it also introduces complexity in analyzing encrypted data. Some encryption methods render data unusable after encryption, limiting their applicability in certain scenarios. Nonetheless, encryption remains a valuable tool in the privacy technology arsenal, particularly as data breaches continue to pose significant risks.
3. Synthetic Data: The Future of Privacy in AI
Perhaps the most exciting development in privacy technology is the emergence of synthetic data. Synthetic data refers to artificially generated data that mimics the properties of real data but does not contain any actual sensitive information. This technology leverages AI and non-AI models to create data that can be used for training machine learning models without the risk of compromising privacy.
Dr. Uzair emphasized the growing importance of synthetic data, particularly in the context of enterprise machine learning. Synthetic data offers a viable solution for organizations looking to balance the need for privacy with the demand for accurate and effective machine learning models. As AI models become increasingly sophisticated, the ability to generate realistic synthetic data has the potential to revolutionize the way organizations handle data privacy.
The technology behind synthetic data has evolved significantly over the past five years, with advancements in deep learning models, such as GANs and transformer models, leading the way. Synthetic data not only protects privacy but also opens up new possibilities for innovation in AI and machine learning.

4. Federated Learning: A Complementary Approach
In addition to the core privacy technologies discussed, Dr. Uzair also touched on federated learning, a technique that allows machine learning models to be trained across multiple decentralized devices while keeping the data local. This approach, used by companies like Google, enables organizations to benefit from large-scale data without compromising individual privacy.
Federated learning is particularly useful in scenarios where data is distributed across different locations, such as mobile devices. By aggregating model updates rather than raw data, federated learning offers a way to train powerful models while preserving privacy.
5. Final Thoughts:
As we continue to see rapid advancements in AI and machine learning, the need for effective privacy technologies will only grow. Data anonymization, encryption, and synthetic data each offer unique benefits and challenges, and understanding their applications is crucial for organizations striving to protect privacy while driving innovation. For data scientists, privacy consultants, and top management, staying informed about these evolving technologies is essential. As Dr. Uzair highlighted, the future of privacy lies in our ability to adapt and innovate, ensuring that we can harness the full potential of AI without compromising the trust and security of the individuals behind the data. When it comes to protecting data privacy, regulations do not define how to do this, that's where it's quite a bit of a gray area. And that's where privacy technologies take the spotlight in terms of remaining compliant with privacy laws and appreciating your users' privacy. So zooming out, in the future, when we talk about AI, the foundations will be built not only on greater quantities of data but also on good or better data.