The demand for data is ever-growing and the recent explosion in the field of artificial intelligence and machine learning has made it clear that without free and fair access to data, there is no moving forward. However, we cannot ignore the importance of individuals’ privacy and confidentiality. This creates a unique dynamic for data consumers and innovators. Without access to high variety, velocity, and volumes of data it is unlikely that models can be trained to give out accurate results. This ultimately affects the general public, especially in the case of a government or state-owned organizations.
Synthetic data fills this gap with artificially produced ‘fake’ data which mirrors the statistical properties of real data. This data can neither be traced back to real individuals nor has any private information making it the safest option to use and share freely and without fear of data breaches and legal consequences.

1. What is Synthetic Data:
Synthetic data is a subset of Generative AI. It is created through Generative Artificial Intelligence (AI) trained on real data, using advanced generative AI models leveraging deep learning techniques that analyze the statistical distributions of original datasets. And generate real-like synthetic data which mimics prominent statistical patterns in the data without memorizing any individual-specific details. The synthetic data generated contains no personally identifiable information (PII), protecting the anonymity and confidentiality of the data sources while maintaining its statistical validity.
2. Why is Synthetic Data Important:
In this world, a business is either data-driven or likely to fail. This is especially true for companies and tech startups that rely on Artificial Intelligence (AI) and Machine Learning (ML) for their product development and growth. As the world looks towards automation, especially in consumer markets, data becomes the single most important factor determining how successful a company will be today and in the future.
However, using real data is a growing liability because of increasing data protection regulations globally. There are more than 130 countries enforcing data privacy laws in the world right now that restrict access, use, and transfer of real data. And the consequences of non-compliance and breaking these laws are severe. Even if you are not breaking any laws, there is always the possibility of a hack or a data leak that can destroy a company’s reputation instantly. According to IBM USD 4.45 million was the global average cost of data breaches in 2023. These risks are not a matter of if but when. So, instead of being reactive, how can companies be proactive about compliance and future-proof their businesses?
Synthetic data eliminates any risk of exposing real or sensitive user information. This means as a Chief Technology Officer (CTO) or a Data Scientist, you can now confidently develop, test, and optimize your algorithms and products while ensuring privacy compliance. For organizations operating in the finance, healthcare, and government domains governed by stringent data regulations, synthetic data enables quick access and easy flow of data while supporting comprehensive testing and validation of systems and training robust AI/ML models without worrying about leaking or handling sensitive information.
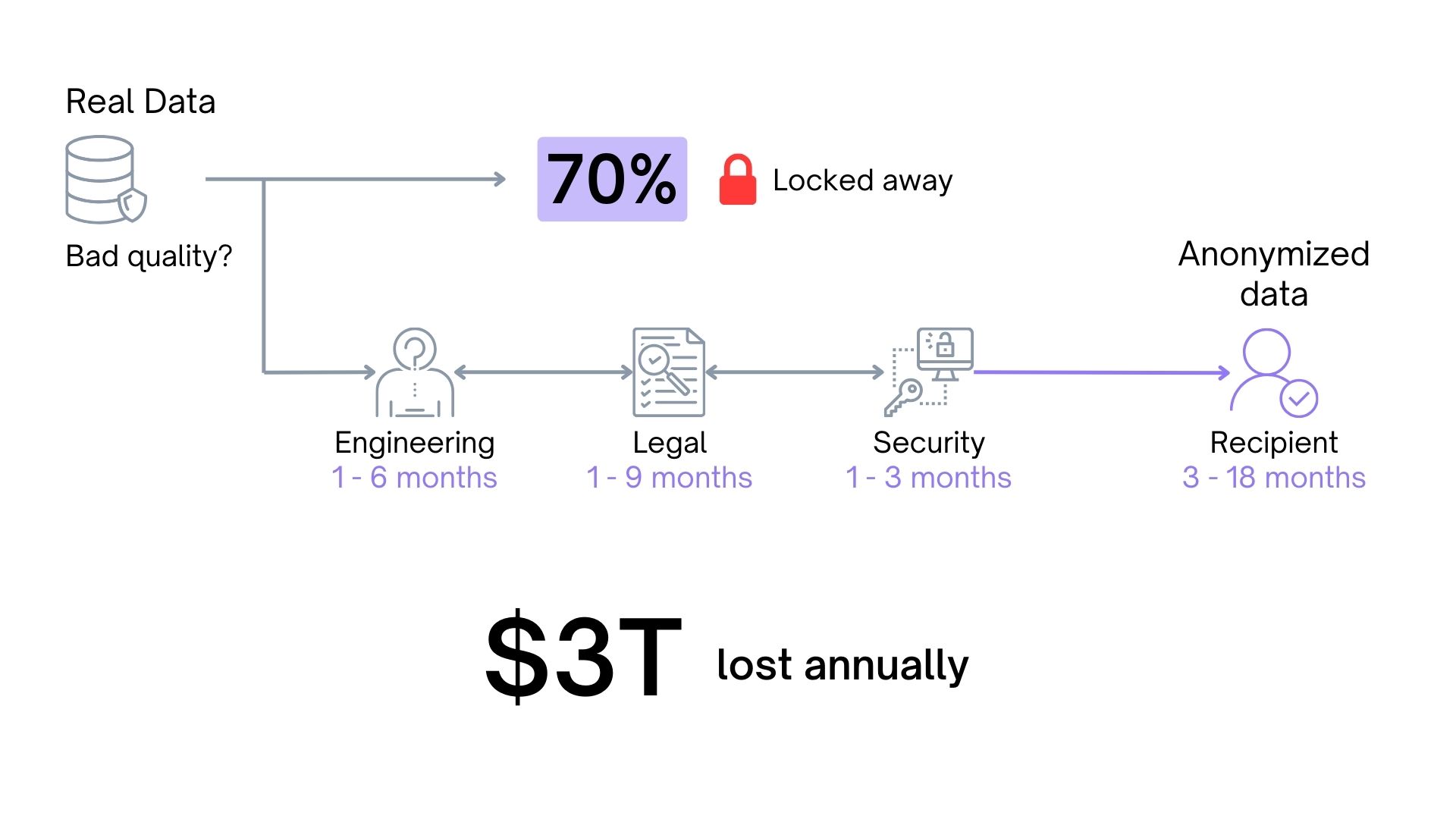
3. Benefits of Synthetic Data:
a. Data Privacy:
With increasing concerns over data breaches and stringent data privacy regulations, synthetic data allows businesses to easily access and share data without compromising the confidentiality of business-sensitive information or the privacy of sensitive real user information. This not only ensures compliance with privacy laws, but also builds trust among users by reducing the risks associated with data breaches, data leaks, and ransomware attacks. As synthetic data cannot be used to infer about any real individual, businesses can use it as a substitute for real data and no longer worry about paying fines for non-compliance with privacy laws globally.
b. Cost-Effective:
Think of a chatbot that isn’t just a chatbot but an AI-enabled assistant that helps consumers pick which color would look better on them. Now think of the amount of data required and the cost to acquire that data. Synthetic data eliminates the need for exhaustive and potentially expensive real data acquisition. Instead, companies can generate diverse datasets through GenAI by utilizing public and private data to generate privacy-preserving synthetic data, or limited datasets to augment data and fulfill different ML performance needs on demand, facilitating cost-effective innovation. This opens up major avenues for startup growth and smaller enterprises looking to compete on a data-driven playing field without the financial burden of large-scale data collection.
c. Scalability and Flexibility:
Unlike real data, synthetic datasets can be easily scaled to meet the demands of various testing scenarios and to interpolate the available real data. Whether simulating user behavior, market trends, or anomalies, synthetic data allows businesses to flexibly adapt to evolving needs without the constraints of limited, pre-existing datasets. This allows organizations to have quick access to reliable datasets that can be used to train AI/ML models in different scenarios as well as build other data-driven solutions.
However, it is worth noting that the random features introduced by GenAI to make synthetic data non-identifiable also shift the distribution away from that of the real data. This means the 'new trends' that your synthetic data might have are not real trends but simulated ones. In short, you cannot create new information from old information. GenAI only solves the operational limitations of less data, not the information-theoretic ones.
d. Bias Mitigation and Fair Algorithm Development:
Synthetic data provides a controlled environment for testing and AI/ML training, enabling companies to mitigate biases that may exist in real data. Such as a larger percentage of a specific gender or ethnicity. This is particularly crucial for industries like finance and healthcare, where fair and unbiased algorithms play an important role in policy development, product innovation, and distribution of funds.
f. Enhanced Collaboration and Knowledge Sharing:
Synthetic data facilitates seamless collaboration among teams by providing a privacy-safe alternative for sharing datasets. This accelerates the pace of innovation as data scientists, analysts, and developers can collaborate without the barriers imposed by data privacy concerns. Synthetic data decreases the time to share data from months to days enabling faster growth at scale.
Read more about advantages of synthetic data here.
4. Types of Synthetic Data:
a. Fully Synthetic Data:
Fully synthetic data is generated entirely by algorithms without relying on real-world data, therefore it has no direct link to real-world individuals making it an effective solution for privacy protection. This high versatility data is generally used for large-scale LLM Models that have specific data needs. Fully synthetic data quality is dependent on the accuracy and robustness of the models used to create it. This data is particularly useful for training machine learning models when real data is sensitive or limited, testing software systems under scenarios not covered by real data, and creating datasets for bench-marking algorithms.
b. Partially Synthetic Data:
Partially synthetic data or hybrid synthetic data, combines real data with synthetic data, offering a balance between data utility and privacy. Partially synthetic data generates synthetic data for certain parts of the dataset while retaining real data points in others, reducing the risk of re-identification by anonymizing sensitive data elements and preserving the utility of the original dataset by maintaining statistical patterns and correlations. Partially synthetic data is useful for replacing sensitive attributes to protect privacy, augmenting real datasets to address class imbalances or test specific conditions, and sharing data for research or collaboration without exposing sensitive information.
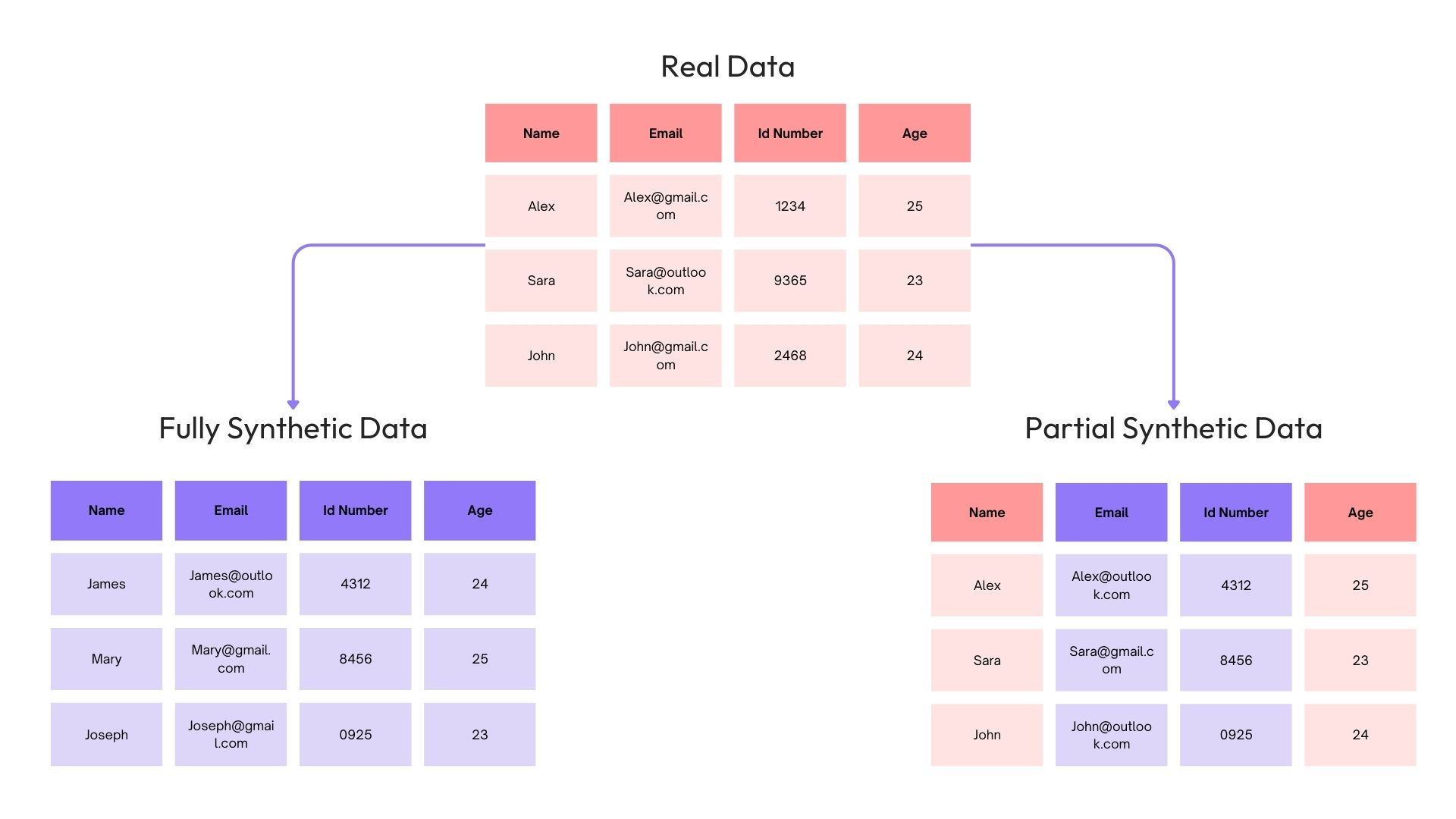
5. Classes of Synthetic Data:
a. Synthetic Text Data:
Synthetic Text Data is artificial textual c:ontent using natural language processing (NLP) techniques, generating sentences, paragraphs, or even entire documents. This data, produced by models like GPT (Generative Pre-trained Transformer) or BERT (Bidirectional Encoder Representations from Transformers), can maintain grammatical correctness and contextual relevance, making it suitable for specific applications like legal, medical, or technical domains. Synthetic text is valuable for training chatbots and virtual assistants, creating large corpora for NLP research, and testing text-based software systems, ensuring that these systems can handle diverse linguistic inputs.
b. Synthetic Tabular Data:
Synthetic Tabular Data mimics structured datasets commonly found in spreadsheets or databases, where columns represent variables and rows represent data points. It preserves the statistical properties and correlations of real data, making it useful for analytical and machine learning tasks. Synthetic tabular data enhances model robustness by providing additional data points for tasks like classification and regression, supports data analysis without compromising privacy, and enables businesses to simulate scenarios and perform what-if analyses for strategic planning.
c. Synthetic Media Data:
Synthetic media data involves the generation of artificial images, videos, or sounds using techniques like generative adversarial networks (GANs). This media closely resembles real-world content, making it useful for realistic simulations and training data. Its applications include training models for computer vision and audio recognition, generating content for VR and AR experiences, and testing media processing algorithms, ensuring these technologies perform effectively across diverse media types.
Read more about types and classes of synthetic data here.
6. Use Cases for Synthetic Data:
a. Finance: Generating synthetic financial transactions to detect fraud without exposing real customer data.
b. Healthcare: Creating synthetic patient records for training medical AI models while maintaining patient privacy.
c. Retail: Simulating customer purchase patterns to optimize inventory management and supply chain operations.
d. Telecommunications: Generating synthetic call data for improving customer service automation without using real communications.
e. Insurance: Modelling synthetic claims data to assess risk and improve underwriting processes.
f. Automotive: Creating synthetic driving scenarios to train autonomous vehicle systems safely and efficiently.
g. Education: Producing synthetic student performance data to evaluate and enhance personalized learning tools.
h. Government: Simulating synthetic census data for policy-making and resource allocation without exposing personal information.
i. E-commerce: Generating synthetic browsing behavior to test recommendation algorithms and enhance user experience.
j. Banking: Using synthetic loan applications to refine credit scoring algorithms and ensure fair lending practices.
Our extensive library of synthetic data use cases exploring different problems across various industries will be out soon.

7. Who is Synthetic Data for:
a. Data Scientists:
Data Scientists can use synthetic data to create effective and efficient data patterns and more accurate AI/ML models using diverse and scalable datasets. By providing a controlled environment that mimics real-world scenarios, data scientists can fine-tune models without compromising sensitive information. This not only streamlines the development process but also enhances the accuracy and robustness of predictive models, ultimately contributing to more informed decision-making.
b. Data Protection, Privacy and Compliance Teams:
For data protection teams synthetic data is a strategic asset that allows them to worry less about what the privacy laws dictate and find ways to meet compliance requirements faster. For example, a use case built with real data needs explicit user consent. With synthetic data, user consent is not needed and therefore, it saves these teams months’ worth of time. By creating artificial datasets that retain the statistical characteristics of real data, data protection teams can ensure that their legal measures are effective, compliant, and adaptable to evolving regulatory landscapes, enabling them to improve their defenses against potential breaches and privacy concerns.
c. Product Developers:
Whether developing software, applications, or advanced technologies, product developers benefit from the flexibility and scalability that synthetic data offers. It accelerates the testing and refinement phases, allowing developers to iterate quickly as all team members can work on the same datasets without worrying about the long approvals required for sharing and accessing data. Synthetic data can be used for rigorous product testing in diverse scenarios without exposing them to the challenges associated with handling real-world sensitive data. This not only expedites the development lifecycle but also mitigates risks and enhances the overall quality of the end product.
d. Data Engineers:
Data Engineers, responsible for the architecture and maintenance of data infrastructure, find synthetic data invaluable in optimizing their workflows. Synthetic data can help in the development and testing of data pipelines, allowing engineers to identify and address potential bottlenecks or vulnerabilities. It provides a controlled environment for troubleshooting and enhancing the efficiency of data processing systems, contributing to the overall robustness of their data infrastructure.
Read more on how synthetic data helps your tam move 10x faster here.
8. Generating Synthetic Data:
Organizations can generate artificial datasets mirroring real-world scenarios by leveraging advanced algorithms and modeling techniques. This process not only ensures privacy compliance but also provides a better alternative to real data. Betterdata has trained algorithms by using state-of-the-art models such as Generative Adversarial Networks (GANs), Diffusion models, Transformers, Variational Autoencoders (VAEs), and Large-Language Models (LLMs) with cutting-edge privacy engineering techniques to generate highly realistic and privacy-preserving synthetic data.
a. Generative Adversarial Networks (GANs):
GANs are deep learning models consisting of two neural networks, a generator and a discriminator, which compete against each other to create realistic synthetic data. The generator produces fake data, while the discriminator tries to distinguish between real and synthetic data, ultimately leading to high-quality data generation.
b. Variational Autoencoders (VAEs):
VAEs are another type of deep learning model used to generate synthetic data. They work by encoding input data into a lower-dimensional latent space and then decoding it back to its original form. This process allows VAEs to generate new, diverse data that closely resembles the original dataset.
c. Simulation-Based Methods:
Simulation-based methods generate synthetic data by simulating complex processes or systems. These methods use domain-specific models, such as physical or mathematical simulations, to produce data that mimics real-world phenomena, often used in fields like finance, healthcare, and engineering.
d. Data Augmentation:
Data augmentation refers to techniques used to increase the size of a dataset by applying transformations such as rotation, scaling, flipping, or noise addition to existing data. These transformations generate new, varied data, often used in image processing and computer vision tasks to improve model generalization.
e. Synthetic Minority Over-sampling Technique (SMOTE):
SMOTE is a statistical technique often used for handling imbalanced datasets. It generates synthetic samples by interpolating between existing instances of the minority class, increasing its representation in the data and improving the performance of machine learning models.
f. Large Language Models (LLMs):
LLMs, such as GPT or BERT, are used to generate text-based synthetic data by learning from vast amounts of textual data. They can be fine-tuned to produce high-quality synthetic text data for various applications, including natural language processing, chatbots, and language translation tasks.
9. Synthetic Data vs Data Anonymization:
Synthetic data is all about generating artificial datasets that look and function like real-world data without containing real user or business-sensitive information. It offers good guarantees for data privacy, data security, data quality, data quantity, and data coverage, making it a very powerful tool for industries working with data.
On the other hand, data anonymization focuses on destroying, masking, or redacting PII data and suppressing non-PII information within a dataset. This method is non-AI based and involves techniques such as pseudonymization, data masking, generalization, data swapping, data perturbation, encryption, tokenization, and generalization to protect individual identities as well as their behavioral attributes.
10. Why Synthetic Data over Legacy Data Anonymization Techniques:
In summary, anonymization techniques operate on this fundamental principle: destroy information to protect privacy and vice versa. Secondly, anonymization is a non-AI-based method, which is why it is not scalable since different anonymization techniques are applied to different data types, have high privacy risks, and are vulnerable to user re-identification risks (As per research about 87% of the population can be reidentified by cross-referencing non-identifiable attributes such as gender, ZIP code, and date of birth). Anonymization also cannot be used to generate more data from limited data. For machine learning, this makes anonymized data useless in most cases since artificial intelligence requires high quality, high quantity, and high diversity data to be successful. Furthermore, legacy anonymization techniques such as data masking, data swapping, k-anonymity, generalization, etc are applied to real data in its raw form. Data scientists have first to spend time and effort in cleaning, and classifying this data, and even after that, the chance of human biases and errors creeping into the dataset is high.
Synthetic data, on the other hand, is an AI method that is scalable as the AI models can automatically understand different data types in a supervised or unsupervised manner. Since synthetic data is artificially generated, it does not belong to real users anymore and therefore, it has low privacy and user re-identification risks. One of the main differentiating factors for synthetic data is the ability to generate more data from limited data. This feature can be used to remove data biases, fill balance missing data sets, and create data for rare scenarios. All these properties position synthetic data as a key technology and the only viable solution to not only address data privacy but also data enhancement and data enrichment challenges faced by data teams and professionals in machine learning.
Read more about why legacy data anonymization techniques are failing here.
11. Synthetic Data for Machine Learning:
On average artificial intelligence needs about 10x the quantity of data points as there are features in your dataset. This means for every 1 column or feature you need at least 10 rows of data, and for a 100 features you would need about 1000 rows of data without which it is unlikely you model will perform as per standards.
As the scale of the model increases so does data quantity reaching millions of bytes of data. This is a lot of data to collect, organize, and refine while adhering to data privacy laws. Another problem is that heavily anonymized data for large ML models is almost useless since artificial requires extensive amounts of unfiltered data. Anonymized data does not provide that as discussed above. Third to keep the model up to date we have to regularly feed new data into the model that covers the latest trends otherwise we risk a data drift.
Synthetic data helps fill this gap with artificially generated data that can be enhanced to cover new trends and fill missing values, remove human biases in the data set, and can be shared safely and quickly with internal and external shareholders since it does not contain any personally identifiable information. This makes synthetic data the only viable and sustainable solution for high-quality model training.
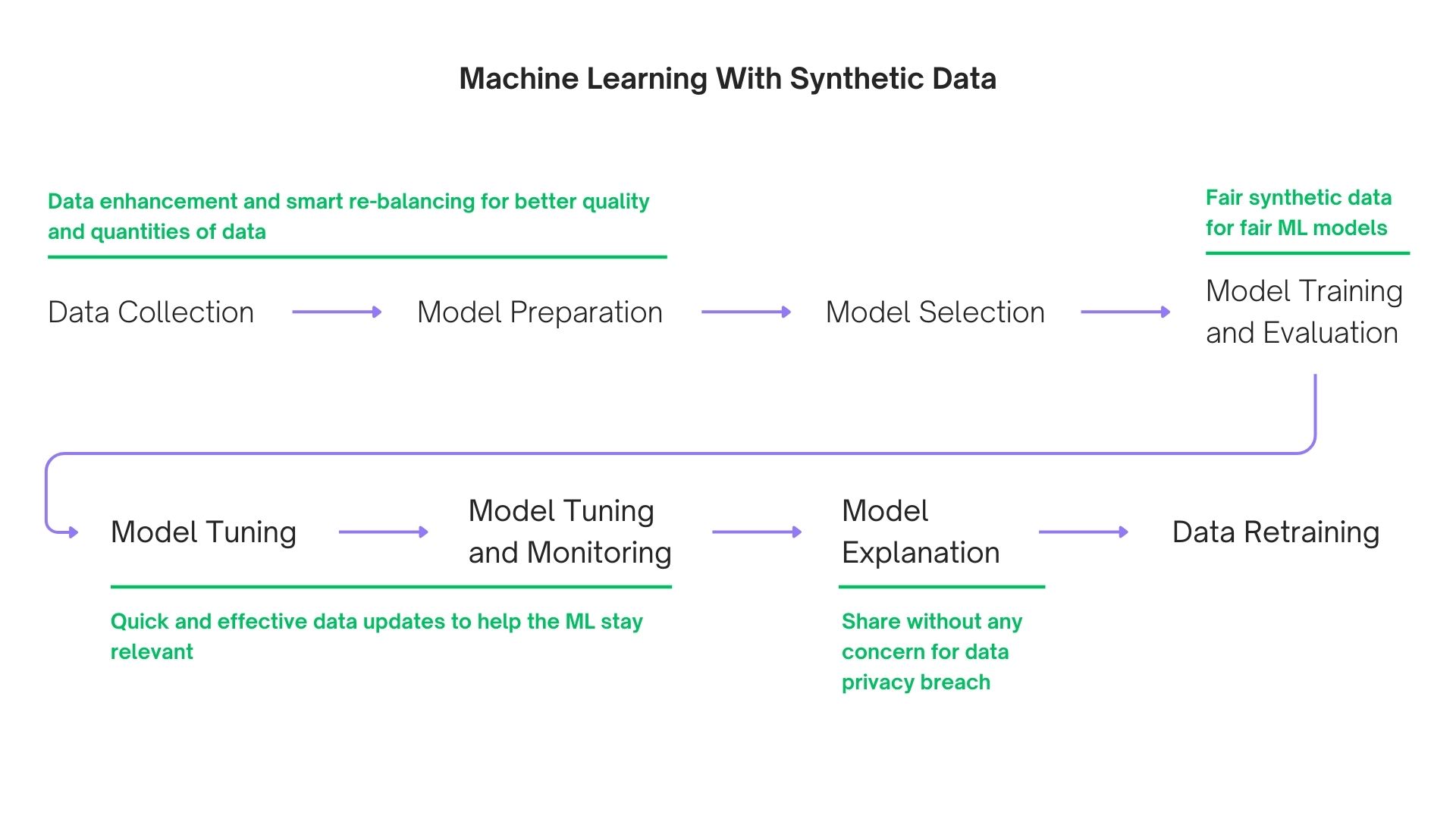
Read more on why synthetic data is the future of AI here.
12. What about Synthetic Data Utility, Accuracy and Privacy:
A general question that is asked is 'can synthetic data be trusted.' Yes it can provided that you fulfil the two core fundamentals of synthetic data generation which determine the utility, accuracy and privacy of your synthetic datasets. These are,
a. Synthetic Data Quality:
Synthetic data quality essentially is analysis of the accuracy of alignment between the synthetic dataset and the real dataset which is determined by the distribution of columns and rows. Column distribution and correlations determine that the synthetic dataset slings with real data and the columns in relation to one-to-one and one-to-all-other align as well. Row quality is concerned with consistency and formulas. Consistency refers to the logical relationship between different rows which if analysed together should make sense. Formulas refer to the statistical relationship between rows which means (especially in the case of finance) that revenue minus costs gives you profit that aligns with the real dataset and maintains a rigid relationship.
b. Synthetic Data Privacy:
Since synthetic data uses Personally Identifiable Information (PII) to generate artificial datasets it is important that we apple to proper mechanism to protect PII. Simply put a sub-standard privacy protection mechanism will cause a lot of leakage which means that real data will be transferred to synthetic data sets. It is also worth noting that traditionally privacy has always had an inverse relationship with data quality. The heavier the legacy data anonymization techniques (masking, encrypting, etc) used the lighter the data utility will be. Synthetic data uses differential privacy to secure PII which allows us to protect data while maintaining high levels of data utility and quality. With closely monitoring data leakage and the chances of an inference attack we can generate 100% secure synthetic datasets.
With monitoring and updating these two fundamentals secure synthetic data can be generated often of higher quality than real data. When you generate synthetic data your receive a report on various metrics that determine the quality, utility and accuracy of synthetic data. Closely monitoring these metrics such as
- Statistical Similarity
- Category and Range Completeness
- Prediction score
- Row Novelty
- Inference
etc. we can ensure that the synthetic data generated is of high quality, utility and privacy.
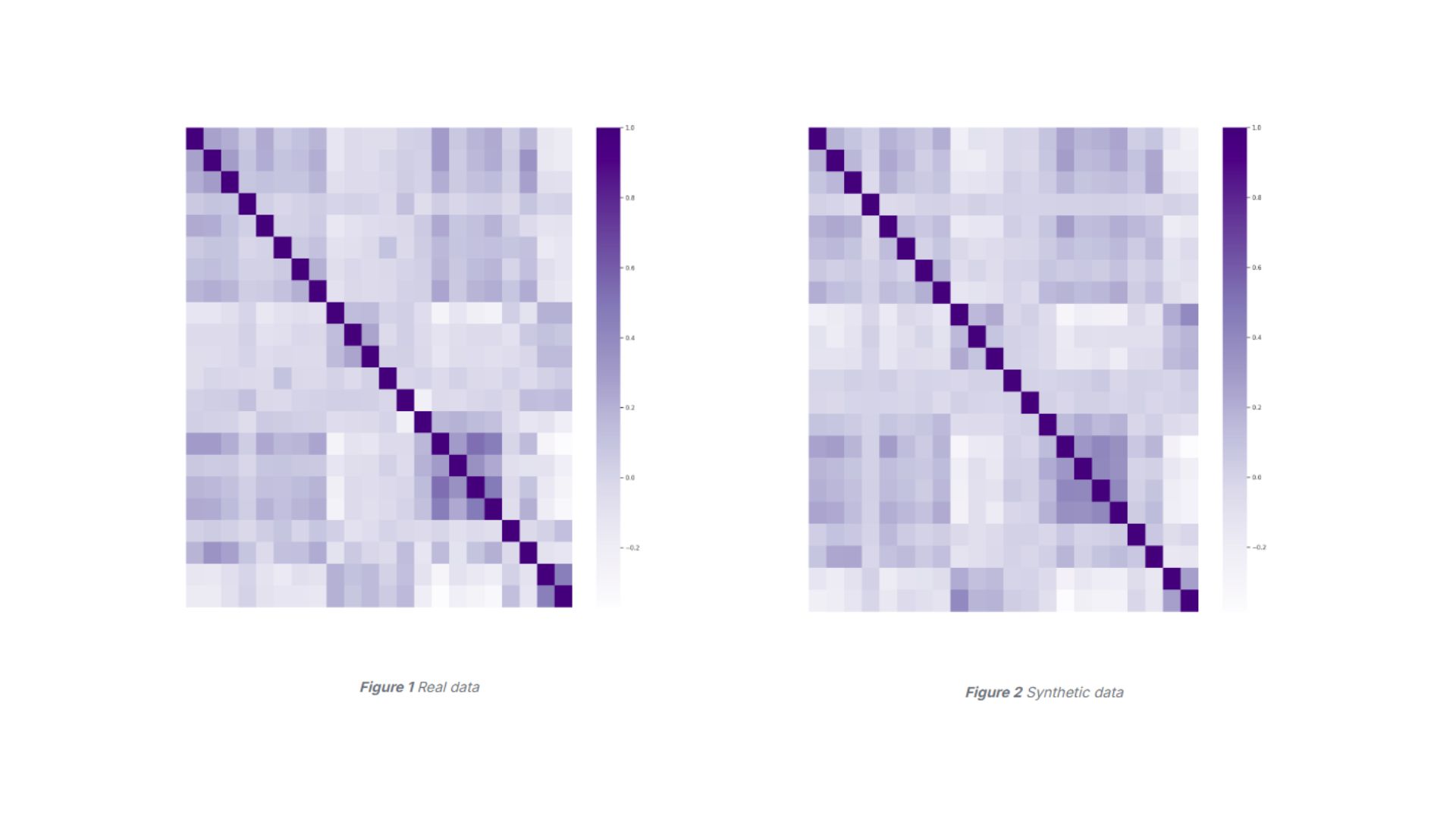
13. Challenges and Risks:
Although synthetic data is poised to solve data privacy and data quality challenges, it comes with its own set of limitations. The key challenges in synthetic data can be divided into four categories explained below:
a. Data Preparation:
This process essentially deals with whether a user can take real data and pre-process it in a way that is fit for input to a synthetic data generation algorithm. This can include steps like automatic detection of data types in a column (Categorical, Numerical, Date, Time, etc.), checking for missing values, inaccuracies, duplicates, and skewness in a dataset, dealing with categorical values, etc. Generally, this process can include the following types of analysis:
- Data type inference
- Data profiling
- Univariate analysis
- Multivariate analysis
- Time-Series analysis
- Text analysis
- File and Image analysis
- Comparing datasets
A key challenge here is how to deal with outliers such that when you have a dataset with a few samples about a certain population, what can be done to avoid the synthetic data generation model from overfitting? The risk here is if a model overfits, it can memorize data instead of learning it and that leads to privacy leakage. Another challenge is how to handle data at scale: how to process a dataset in a distributed way, whether to use binary formats (Parquet, HDF5) or non-binary formats (CSV, Excel), should the entire dataset be loaded into memory first or can it be processed as it is read in a streaming fashion. The risk here is subject to business requirements as it may be difficult to generate synthetic data at scale if data cannot be prepared for the synthetic data generation algorithm.
b. Data Generation:
This process deals with the generation of synthetic data. For this process, an AI algorithm is required based on your metadata. Next, using real data, the model is trained which can take a few minutes to a few hours to a few days, subject to compute resources a user has. In the training phase, the model will learn patterns from the real data and once training is finished, the model can be used to generate synthetic data artificially from scratch. A key challenge here is how to avoid the model from overfitting and the associated risk is that if the model overfits, it can generate real data samples as synthetic output.
c. Data Evaluation:
After synthetic data is generated, it can be compared with real data on three bases:
- Data fidelity: this explains how similar synthetic data is in distribution to real data
- Data utility: this explains how ML models perform trained on synthetic data vs. real data
- Data privacy: this explains if any record from synthetic data output can be traced back to a real individual from the real data used for training
A key challenge here is there is no unified evaluation framework for synthetic data and as such, it is difficult to quantify data privacy and data quality guarantees. The risk associated with it is that without effectively proving how good synthetic data is, its adoption can be limited.
d. Stakeholder Alignment:
Data teams typically work hand in hand with business units and legal teams. The challenge here is natural: data teams are technical and demand high data quality guarantees from synthetic data, whereas legal teams are non-technical in nature and demand high privacy guarantees from synthetic data. This creates a dilemma on whether to optimize synthetic data for quality or privacy, as doing so for both is just simply not possible. The associated risk here is since there are no concrete guidelines on using synthetic data for different applications, business owners are skeptical of it and resist its value propositions.
14. Summary:
As the world moves towards digitization, automation, AI, and augmented reality, synthetic data presents itself as an enabler; creating and supporting the free flow of high-quality data among organizations to be used for AI/ML, Business Intelligence (BI), data licensing, product testing and development, policy development and so much more without having to worry about non-compliance with data protection regulations. With synthetic data, businesses can minimize the potential risks of lawsuits and brand reputation. It opens up new avenues for innovation and collaboration among data professionals, teams, and organizations alike, fostering huge growth potentials and use cases that were just not possible before.