1. What is Synthetic Data?
Synthetic data is artificial data generated with purpose-made deep generative models such as GANs and LLMs to obscure direct identifiers while maintaining data utility at a granular level. Because synthetic data mimics the statistical patterns of source data and omits PIIs, it cannot be traced back to real individuals making it the most secure option to use and share data freely and quickly. This creates numerous opportunities for enterprises to apply synthetic data to different use cases across industries. Essentially what an enterprise would but cannot achieve with real data, it can do so by using synthetic data.

2. How do you generate synthetic data?
Synthetic data is generated by training Deep Generative Models (DGMs) on real data, allowing them to learn its statistical properties and distributions. These models then produce synthetic data that looks, feels, and functions like the original data. Organizations use a range of technologies for synthetic data generation such as ARFs, VAEs, GANs, and LLMs, all with the shared goal of creating high-quality data generators capable of generating realistic synthetic data that closely mirrors real data.
However, synthetic data alone does not inherently provide privacy guarantees. Embedding differential privacy within these models is essential to ensure measurable privacy protection while rigorously tracking data privacy metrics like record repetition and membership inference score, depending on your synthetic data use case.
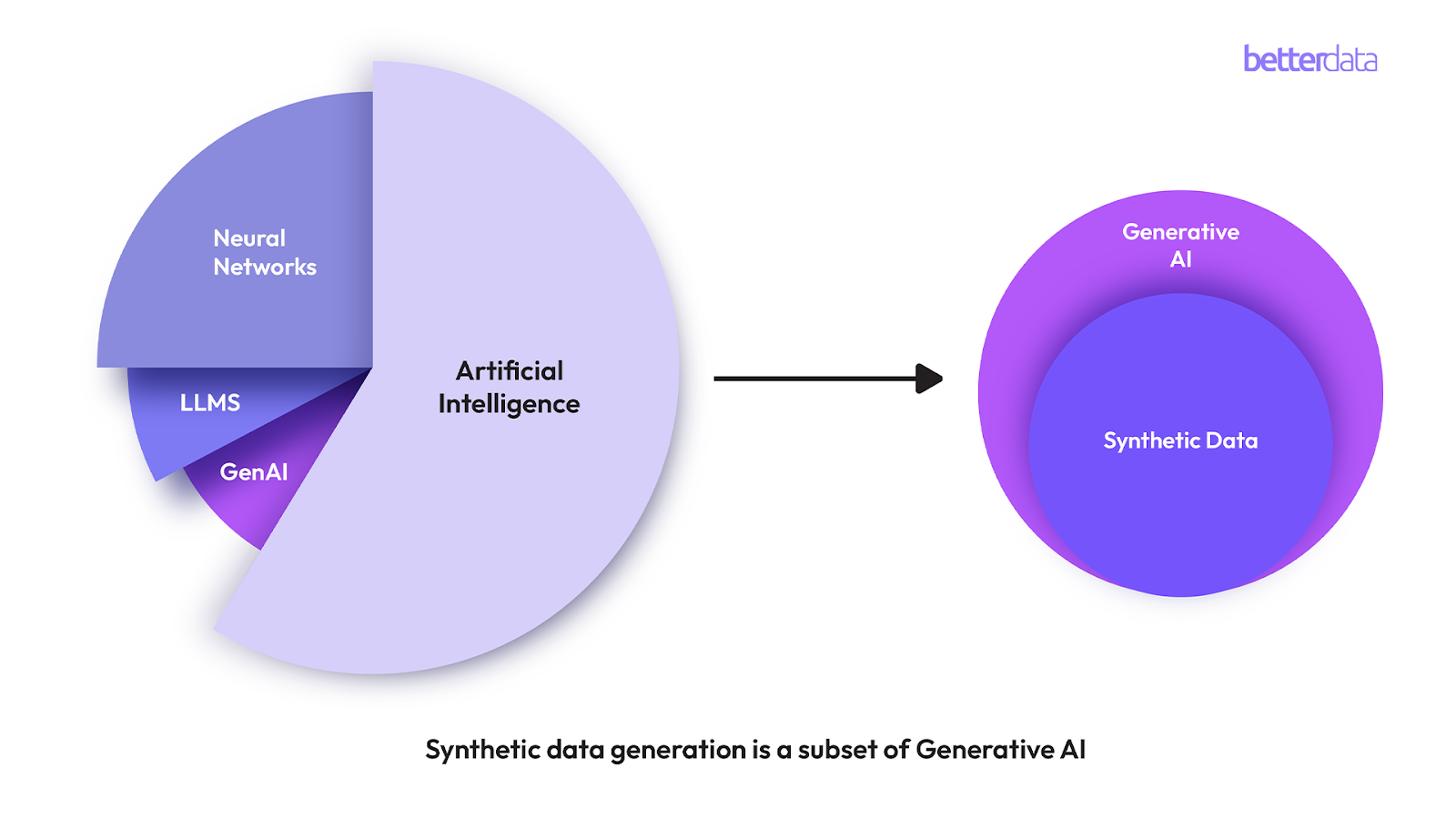
At Betterdata, we have been pioneering SOTA DGMs with privacy engineering to produce high-quality synthetic data that is also private. Contact us to explore how we can support your data needs.
3. Benefits of synthetic data:
a. Protect Data Privacy:
Synthetic data generated through robust ML models and with excellent privacy measures installed is 100% anonymous and as such does not contain any reidentification risk. Contrary to the legacy data anonymization techniques which have reidentification risks. This places enterprises in a position where they do not have to to worry about legal action and hefty fines when using or sharing synthetic data.
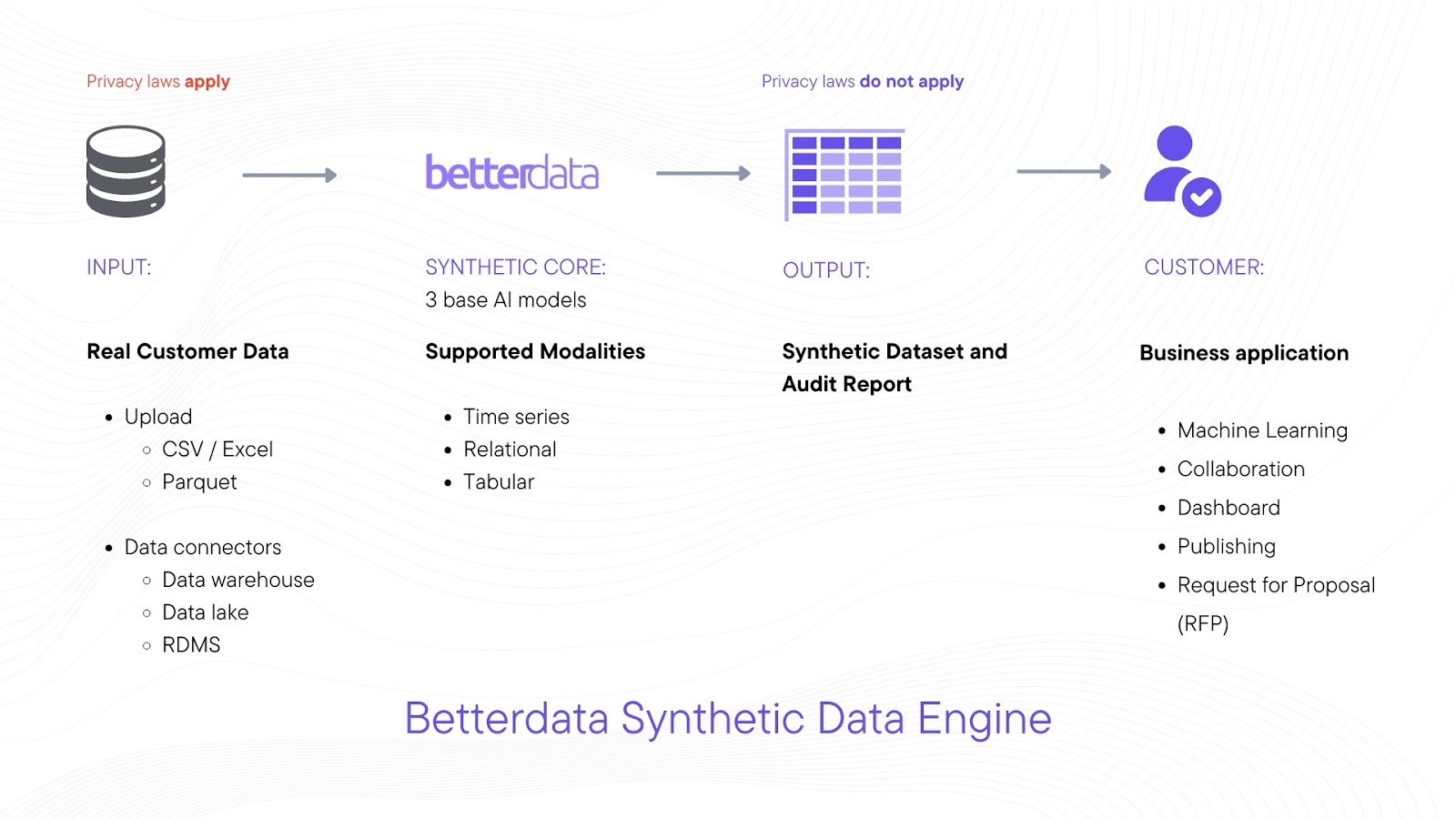
b. Fair AI/ML Development:
Real data is biased, imbalanced, and often incomplete. Synthetic data generators can fix these core data problems for fair and robust AI/ML model development. Synthetic data removes biases, balances data, and fills in missing information based on learnings from training data.

c. Maintain Data Utility:
Data is either private or useful. It can’t be both. Synthetic data in essence is a non-identifiable substitute for real data which contains all its statistical properties and attributes and, as such is not private. This ensures a high level of data utility since data teams get a comprehensive view of collected data for analysis. In contrast, legacy data anonymization has an inverse relationship with data utility since these techniques encrypt, destroy, or suppress data causing data utility to decrease and hence can't be used in majority use cases especially machine learning and analysis.

d. Data Monetization:
A synthetic data use case in itself, enterprises with extensive data collection can bypass data privacy laws using synthetic data when commercializing such data opening new opportunities for revenue generation through data monetization.

e. Share Data Freely:
Enterprises can share synthetic data with internal and external stakeholders much more faster and securely than anonymized data. Synthetic data does not require enterprises to apply privacy techniques repeatedly to hide and display information based on the team or person the data is being shared with nor does it require intense scrutiny from privacy teams. Once generated with adequate privacy measures it can be shared and used much more freely without risking sensitive data exposure.
4. Use Cases for Synthetic Data:
a. Banking and Finance
- Fraud Detection: Synthetic transaction data enables models to detect fraudulent patterns without exposing sensitive customer information.
- Credit Scoring: Helps train algorithms on simulated financial histories, improving scoring models without accessing real financial data.
- Risk Modeling and Stress Testing: Enables banks to create synthetic scenarios to stress-test portfolios against market volatility and regulatory requirements.
b. Healthcare and Life Sciences
- Clinical Trial Simulation: Creates synthetic patient populations to test and refine clinical trial designs, reducing time and cost.
- Medical Imaging: Provides high-quality synthetic images (e.g., X-rays, MRIs) to train diagnostic models, preserving patient privacy.
- Predictive Patient Health Monitoring: Simulates patient data to predict disease progression and outcomes, useful for developing AI-based patient monitoring systems.
c. Retail and E-commerce
- Customer Behavior Modeling: Generates synthetic customer journey data to analyze buying behaviors, enabling personalized recommendations.
- Inventory and Demand Forecasting: Simulates product demand patterns across locations to improve inventory management and reduce stockouts.
- Pricing Optimization: Uses synthetic data to test and optimize dynamic pricing algorithms for different demographics and demand scenarios.
d. Telecommunications
- Network Optimization: Creates synthetic data on network traffic to optimize load balancing and improve service quality.
- Customer Churn Prediction: Simulates customer behavior to predict churn and devise retention strategies, avoiding real customer data.
- Anomaly Detection in Network Security: Generates synthetic data for unusual traffic patterns to train AI systems for identifying and responding to network threats.
e. Insurance
- Risk Assessment and Policy Pricing: Simulates customer profiles to refine risk models, enabling accurate policy pricing without disclosing real customer data.
- Claims Fraud Detection: Provides synthetic claim data to train fraud detection algorithms, reducing exposure of real claimants' information.
- Predictive Claims Management: Enables synthetic scenario testing for managing claim costs and processing efficiency.
f. Automotive and Autonomous Vehicles
- Autonomous Driving Training: Generates synthetic driving scenarios (weather conditions, pedestrian behaviors) to train self-driving car algorithms.
- Sensor Calibration and Testing: Provides synthetic sensor data for camera and radar calibration, improving the accuracy and reliability of autonomous systems.
- Vehicle Safety Simulation: Enables crash and accident simulations for safety testing without real-life testing constraints.
g. Education
- Student Performance Prediction: Uses synthetic data to model student performance across demographics, improving educational interventions.
- Personalized Learning Systems: Simulates diverse learning paths to train AI for adaptive learning platforms, enhancing personalized education.
- Assessment Development: Provides synthetic data for exam scenarios to improve assessment tools, ensuring fairness and adaptability in testing environments.
h. Government and Defense
- Cybersecurity Training: Generates synthetic cyberattack data for training defense systems to detect and respond to attacks effectively.
- Mission Simulation and Planning: Enables simulation of military missions and strategic operations to refine plans and optimize logistics.
- Public Policy Testing: Simulates demographic data to test public policies before implementation, ensuring they’re effective and equitable.
i. Marketing
- Customer Segmentation: Generates synthetic profiles to refine customer segmentation strategies, helping target ads more effectively.
- Ad Campaign Performance Simulation: Simulates different demographic responses to test ad campaign effectiveness across target groups.
- Sentiment Analysis Training: Provides synthetic customer feedback for training NLP models on sentiment analysis, improving brand monitoring tools.
j. Manufacturing and Industry 4.0
- Predictive Maintenance: Uses synthetic machine data to predict equipment failures, reducing downtime and maintenance costs.
- Quality Control and Defect Detection: Generates synthetic images of defective products to train quality control AI without needing real-world defects.
- Supply Chain Simulation: Creates synthetic data to optimize supply chain logistics, accounting for demand fluctuations and potential disruptions.
5. The Future of Data-Driven Innovation:
Synthetic data is the potential of real data unleashed. The commercialization of AI, for example, is based on abundant amounts of data being collected daily. However, one massive challenge in AI development is the accuracy of its models, which are often biased and inaccurate. Amazon's AI recruiting tool was discontinued for this specific reason—it showed bias against women. Other examples of bad AI due to bad data are,
- Carnegie Mellon University found Google’s ad system was gender biased, showing high-paying job ads to men more often than women.
- ProPublica’s 2016 investigation showed racial bias in COMPAS, labeling Black defendants as having higher reoffending risks than white ones.
This happens because models learn from data and so do humans. Incomplete, biased, and partially hidden data leads to inaccurate model training. With humans, it comes down to guesswork where data teams, analysts, etc. are forced to make assumptions from data that only give you the full picture. As enterprises adopt data-driven strategies to drive growth and innovation, synthetic data offers better data for accurate and precise analysis, decision-making, model training, and so on, whatever the use case might be.